Articles
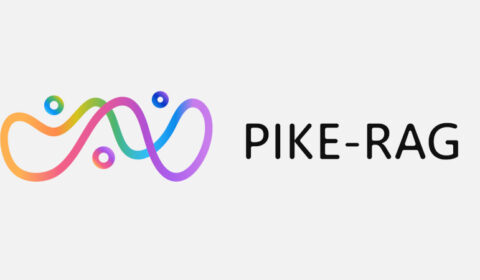
A key challenge, and opportunity, of large language models (LLMs) is bridging the gap between their training data and the vast amount of unfamiliar information they encounter in real-world applications. Successfully navigating this divide could unlock a new era of…

TimeDP:创新时间序列生成模型,突破跨领域应用局限性
人工智能技术的持续演进,让时间序列数据在众多领域中的应用价值愈发凸显,尤其是在医疗健康、金融市场、气象预测和交通管理等行业。时间序列生成技术不仅可以弥补数据的不足,还能在无实际风险的环境中进行模拟和预测,因此具有重要的实际应用意义。 例如,在医疗领域,生成高质量的时间序列数据可以在保护隐私的前提下,帮助解决数据的稀缺问题,为疾病预测、个性化治疗等提供更加丰富的数据支持。金融行业则可以利用生成的市场...
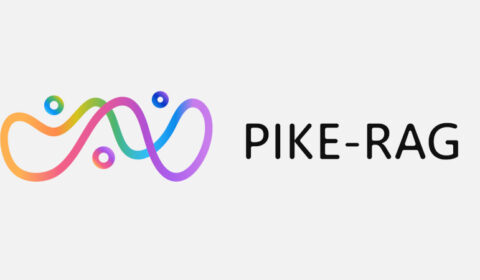
PIKE-RAG:解锁领域私有数据价值,推动LLMs工业应用落地
编者按:随着人工智能技术的快速发展,检索增强生成(RAG)系统已成为扩展大语言模型(LLMs)能力的重要手段之一。然而,当这些系统应用于复杂多样的工业场景时,仍面临诸多挑战,尤其是在处理领域特定知识和复杂推理任务时。对此,微软亚洲研究院的研究员们提出了 PIKE-RAG,通过多层次异构知识库构建、任务驱动的系统搭建策略以及自我进化的领域知识学习机制,显著提升了 LLMs 在复杂工业场景中的推理和应...
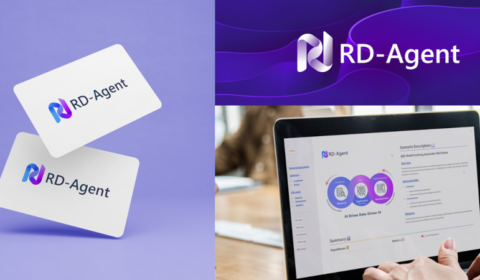
In industry today, research and development (R&D) plays a pivotal role in boosting productivity, especially in the AI era. However, the rapid advance of AI has exposed the limitations of traditional R&D automation methods. These methods often lack the intelligence…

Since the Industrial Revolution, the burning of fossil fuels and changes in land use, especially deforestation, have driven the rise in atmospheric carbon dioxide (CO2). While terrestrial vegetation and oceans serve as natural carbon sinks, absorbing some of this CO2,…
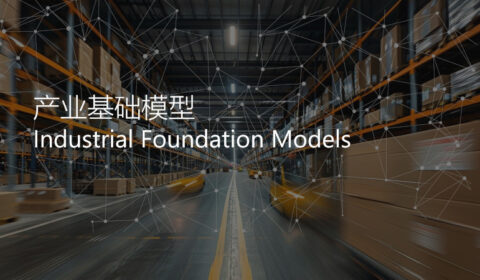
Although large language models (LLMs) excel in language-focused tasks like news writing, document summarization, customer service, and virtual assistants, they face challenges when it comes to learning and inference on numeric and structured industry data, such as tabular data and…
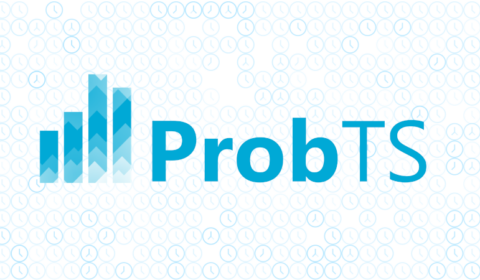
Author: Machine Learning Group Time-series forecasting is crucial across various industries, including health, energy, commerce, climate, etc. Accurate forecasts over different prediction horizons are essential for both short-term and long-term planning needs across these domains. For instance, during a public health…