Microsoft Foundation Models for Health and Life Sciences
Revolutionizing Healthcare with Cutting-edge AI Solutions
Microsoft and our partners, have made significant investments in the research and development of multi-modal models specifically designed for healthcare and life sciences. Our commitment to innovation is driven by the goal of transforming these critical fields through the implementation of advanced GenAI. See the full catalog of models here: https://aka.ms/health-life-sciences (opens in new tab)
Innovative Multi-Modal Models
Microsoft and partner foundation models are built from the ground up using vast medical datasets, and are ready for fine-tuning to address the unique challenges of healthcare and life sciences. These models are capable of processing and analyzing diverse types of data, including medical images, patient records, and genomic information, to provide comprehensive insights and support decision-making.
The versatility of our models allows them to be deployed in a range of scenarios. From enhancing image quality analysis to predicting patient outcomes and identifying potential treatment pathways, our models are designed to support healthcare professionals in delivering better patient care. In life sciences, these models may facilitate groundbreaking research and accelerate the development of new therapies and medical solutions.
Microsoft Multi-Modal Models | Select models summary
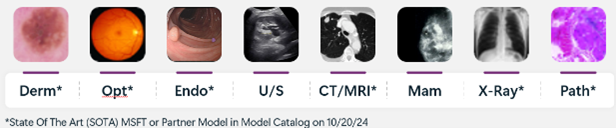
Microsoft has made development quick starts available (opens in new tab) for the below, and other select models.
MedImageInsight is an advanced embedding model for medical image analysis. It supports tasks like classification and similarity search, helping streamline workflows in various medical fields such as radiology, pathology, ophthalmology, and dermatology. The model can be used to develop tools for automatically routing scans to specialists or flagging potential abnormalities, quality analysis, improving efficiency, and patient outcomes. It also supports responsible AI practices like out-of-distribution detection and drift monitoring to ensure stability and reliability in dynamic medical imaging environments.