AI for Precision Health: Learning the language of nature and patients | Microsoft Research Forum
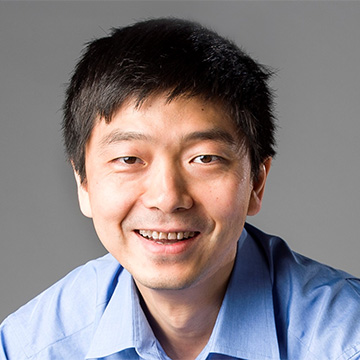
Hosted by Hoifung Poon, with Lili Qiu, Ava Amini, Carlo Bifulco, and Matthew Lungren at Microsoft Research Forum, Episode 5
This panel discussion with Microsoft researchers and external guests explores the transformative potential of generative AI in learning the language of nature and patients for precision health, from proteins to medical imaging, from electronic medical records to home health monitoring. Emphasis is placed on the end-to-end innovation cycle from foundational research to deep partnership to productization.
-
Microsoft research copilot experience How is AI catalyzing a shift towards precision health care, and what are the key challenges and opportunities in this transformation?
Transcript: Panel Discussion
AI for Precision Health: Learning the language of nature and patients
Hoifung Poon (host), General Manager, Microsoft Research Health Futures
Lili Qiu, Assistant Managing Director, Microsoft Research Asia
Ava Amini, Senior Researcher, Microsoft Research Health Futures
Carlo Bifulco, CMO, Providence Genomics
Matthew Lungren, Chief Scientific Officer, Microsoft Health and Life Sciences
This panel discussion with Microsoft researchers and external guests explores the transformative potential of generative AI in learning the language of nature and patients for precision health, from proteins to medical imaging, from electronic medical records to home health monitoring. Emphasis is placed on the end-to-end innovation cycle from foundational research to deep partnership to productization.
Microsoft Research Forum, February 25, 2025
WILL GUYMAN, Group Product Manager, Healthcare AI Models: To talk about generative AI models and the impact they are beginning to have in the real world, Hoifung Poon will host a panel discussion with a distinguished group of experts. Representing our Microsoft Research is Ava Amini and Lili Qiu. They are joined by Microsoft’s Chief Scientific Officer in Health and Life Sciences Dr. Matthew Lungren, who is also a practicing physician and maintains research and teaching roles at Stanford University in AI and medicine. To round out a stellar panel, Providence’s Chief Medical Officer Carlo Bifulco joins to provide another voice on the real-world implementation of generative AI in clinical settings. Back to you, Hoifung.
HOIFUNG POON: Hi, everyone. Welcome to Microsoft Research Forum. Today we will have a very exciting panel discussing AI for Precision Health. As we all know, there are two fundamental challenges in today’s health care, right? So first, medicine can be very imprecise and doesn’t work for the patient, right? So for example, immunotherapy is the cutting edge cancer treatment today. And indeed, you will have some of the blockbuster drug like Keytruda? that can, you know, work miracles for some of the late-stage cancer patients. But overall, the survival is still hovering around 20, 30%, right. And then second challenge is like high-quality healthcare is just too expensive and very hard to scale. Right. So, for example, even in the U.S., 85% of cancer patients, actually are treated in this, rural or community hospital that simply doesn’t have the kind of resources and care quality like some of those more comprehensive cancer center like Mayo or Memorial Sloan, right. So and, sometime disparities in you know, simple information access, can be life or death. So what’s really exciting, these days, is that the GenAI revolution really brings us unprecedented capability. To start, … you can think about it as learning the language of nature to patients, right? And that starts to give us some very powerful tools to start accelerating biomedical discovery so we can drastically improve the healthcare quality and also, career opportunity for us to drastically reduce our healthcare costs. So now we can democratize that high-quality healthcare for everyone.
So now, of course, this undertaking is a really, kind of moonshot aspiration and really takes way more than a village. Right? So at Microsoft Research, we are super blessed with the privilege to actually have fostered deep collaboration across amazing researchers all around the globe and also with our, kind of, beloved health and life sciences product division.
And also we have key external stakeholders, such as large healthcare systems. So, you will see that, this panel really is a perfect reflection of that deep collaboration across the board. So, we will start with a quick intro by every panelist about who they are and what’s the chatter and what gets them excited these days. And also we’ll dive deep into the key opportunities and challenges. So, maybe Ava, can we start with you?
Ava Amini: Absolutely. Yeah. My name is Ava Amini, I’m a senior researcher at Microsoft Research, based in the New England Lab, and also working closely with Health Futures. My passion is really in building new AI methods to accelerate our ability to understand biology and also to design biology. And this is rooted in kind of a fundamental curiosity about bringing the power of computation and quantitative science and engineering to the biological world so that we can learn the language at which biology operates. And for me personally, I’m most interested in how behavior at the cellular level arises from the interactions and activity of individual biomolecules, how these processes become dysregulated in disease, and how we can understand those regulations and dysregulations to now develop more effective and more personalized treatments. So really thinking about the vision of bringing the power of AI to unlock new biological insight so that we can move towards our ability to create new therapies and better interventions that hopefully could be implemented in the clinic and at the patient level.
Poon: Well, that’s fascinating. Like you are learning the language of biomolecules and so far, and really the fundamental kind of biology, So Lili, do you want to go next?
Lili Qiu: Sure. I’mLili Qiu, Assistant Managing Director, Microsoft Research Asia Microsoft Research Asia, mainly responsible for leading the Shanghai Lab. Our mission is to develop a sensing and machine learning for healthcare. Complementary to Ava, our focus is to learn the language our patients outside hospitals. With the rise of chronic diseases, unpredictable health crisis and the demand for personalized treatment, traditional hospital-based monitoring is no longer sufficient.
Consider a patient with a cardiovascular killer disease who visits hospital just a few times a year. On the surface, everything seems fine; stable blood pressure, a steady heart rate, and normal respiration pattern. Yet, between these breaks, subtle flucuations can occur. Warning signs that remain invisible, until they escalate into life-threatening emergencies. For me, this scenario isn’t an abstract concept, but a painful reality. I lost both my mom and my grandmom to cardiovascular disease while they were at home. Their passing revealed how critical it is to have better monitoring tools that extend beyond hospital wards. Their memory was what motivated me to explore and develop in-home continuous monitoring tools to prevent similar heartbreaking loss and protect patients even when they are not at hospital.
Advancements in sensing and I could make it possible to track individuals’ health in real time by continuously monitoring vital signs and body movement. Health care providers can gain a deep and more comprehensive and accurate understanding of a patient’s condition. This could yield many benefits. Here I just will highlight three of them: First, we could do early detection and prevention. With continuous monitoring deviation from normal health parameters can be detected early, allowing for timely intervention before a condition gets worse.
Second, personalized and adaptive treatment plans is possible. No two patients at the same. Precision medicine requires individualized treatment plans based on real-time data. Continuous monitoring allows doctor to tailor their medication and lifestyle recommendation and therapy strategies based on actual patient behavior and physiological response over time. Moreover, we could enhance the patient engagement and quality of life while reducing healthcare costs.
When patients are equipped with real time insight into their own health, they become more engaged in their well-being. Wearable devices and mobile apps to empower individuals to make informed lifestyle choices, adhere to treatment plans, and actively participate in managing their health. This could not only improve patient outcome, but also significantly cut down healthcare costs.
Poon: Awesome. Well, that’s, I really appreciate you sharing some of those, kind of really powerful personal stories, right. So, that actually remind me so many years ago, one of my colleagues, share also a very kind of, like, inspiring story. She had, brother, right, who was diagnosed with cancer, in actually Eastern Washington, Spokane, specifically.
And they found a mutation that actually renders standard healthcare doesn’t work. And so normally the prognosis is like six months, right. So and, and my colleague persuaded him to literally drive two hours west to for a hutch. And there they found a matching trial that actually put the cancer in remission, right. So that that kind of, sort of really powerful story is like, how can we actually democratize that kind of, right, to everyone so that it, it doesn’t just, so on that. On that note, actually, Carlo, do you want to go next? Right. Because you are so really deep in the trenches.
Carlo Bifulco: Yeah. Thank you, Hoifung, indeed in the trenches. Yeah. So my background is, physician scientist, currently serving as CMO of Providence Genomics. For people unfamiliar with Providence, big healthcare network on the West Coast. And our program’s focus is really to bring precision medicine and personalized medicine to cancer patients in the context of that, kind of, very diverse network. On the research side, my work is really focused on genomics, but also spatial biology and AI. And the approach is really translational. I think we try to bring all these modalities to impact patient care in the future. And current patient care whenever we can. Specifically, for this, I think we I had the fortune of working with you all from the Microsoft research team now, I think for six years. And I think we have so much convergence. Right. I think we had a very interesting applications of NLP and AI in the real-world evidence kind of space, clinical trial matching. And we have built an ecosystem which extends to precision medicine to solutions like mobile network tumor boards and other things that really, hopefully, impact patient care.
I think most of what I’m most excited currently is really, you know, an opportunity we had recently which is focused on making a paradigm shift in pathology. We brought generative AI and large foundation models to that field. I think we were one of the first groups to do so, with a very large, open-weight model called Prov-GigaPath.
I think it’s approximately, you know, more than 1 billion parameters, 1.3 billion, if I remember correctly. You know, very impressive, we had 500,000 downloads on Hugging Face. So, super surprised by the impact. And, you know, very pleased by the impact that this is having. And for the future, you know, working on integrating all these modalities, multimodalities hopefully, with things like genomics, spatial biology and so forth.
Poon: Yeah. Thanks so much, Carlo. Obviously a disclaimer is that we have this, super privilege to be able to work with you, right. Exactly. Like when I do the math, yes years. Sorry. And oftentimes like, the challenge is like, yes, so we are all machine learning and modeling folks, right? And then we often think of our machine learning and modeling, but also there is lots of groundwork, right? How do we actually get the data, get the compute set up. Also, adhere to privacy compliance, make sure everything is responsible. And then having this super privilege to be able to really deeply collaborate with you. And also I think that also leads to a very exciting prospect, is that how can we kind of take the learning from, like, this, some of this, kind of, deep partnership, right, and really try to start to impact? Can we start to impact millions of, if not billions of patient, rights? And for that we need scaling.
So, Matt, no pressure, but really want to, get your perspective here.
Matt Lungren: It’s all about scale, Hoifung. So, I’m Matt Lungren, I’m the chief scientific officer at Microsoft Health and Life Sciences. And I’ve kind of got an interesting role. So my background as an academic physician, and, you know, spent a lot of time sort of in both the clinical world, but, you know, also in the core research world, right. And sort of bridging those gaps, kind of starting to become a pattern, I guess, in my career. But now, I kind of do the same thing. But to your point, Hoifung, it’s definitely a scaled effort. And so, we are a group of data scientists, PMs, and obviously some engineers that really are focused on working bidirectionally across both the organization and externally with partners. And what we’re looking to do is we’re saying, okay, what are the core problems in health care? Where are the gaps in some of the technology that we see today? And then what kind of innovations are happening that might, you know, fill those gaps? But the bidirectional aspect is also kind of translating some of those gaps or opportunities in the healthcare space over to some of the core innovation areas to say, hey, I think if you know, if you had if you had a technique that could actually address this, we can actually make a huge difference. And I think, I think what’s really wonderful about working in sort of this collaborative group as a great example is you’ve heard sort of from the biologic discovery, the sort of clinical translational, you’re hearing about how genomics and images kind of come into play.
There’s sort of a beautiful story here about all of these modalities kind of coming together, to your point, Hoifung, to learn the language of life or learn language biology. And I think we’re in a really interesting moment where, you know, we recognize that these powerful language models are, you know, they’re very competent across a lot of different concepts, and particularly in the tech space. And where we see the opportunity is really to translate that ability to, you know, easily access the information, process the information, potentially even enrich the information. But also leverage the data that we know has a lot more information about our patients. And that’s obviously clinically, in the imaging space. And so, I’m just delighted about the opportunities to come. And again, I think this effort that we’ve talked about, the model that the Carlo referenced is currently on the Azure catalog across many other modalities as well. And really unlocks the opportunities for the healthcare developer ecosystem to say, hey, you’ve actually got me pretty close to my goal line here with some of the things you developed, let me take it to the finish line and start to translate that at scale. And that’s exactly what we’re seeing.
Poon: Yeah, thanks. Thanks a lot, Matt. And really, amazing to see your leaderships and, and try to actually get a lot of this kind of, you know, models, right, to start being able to democratize them in AI foundries and so far to really start to put the put them in the hands, right, of folks who can start playing with those model and explore applications. So super, super exciting. So, so one theme I’m really kind of hearing and seeing is like, there is, sort of like a lot of this kind of challenges, as Matt, you just mentioned. Like in the text modality, we kind of learn relatively, quite deeply. Right. But, on the other hand, there are many biomedical modality that are non-text modality that seem to have a lot of unique challenges. So Ava, I want to kind of get back to you is like you, you and the team have done some really amazing work, right? As you mentioned, on biomolecule proteins and so forth. So, what’s your top of mind in terms of some of the key challenges that you see? And what are some of the learning you have found?
Amini: Yeah. I think for me, the what’s really top of mind connects back to this theme of bidirectionality that Matt and Carlo and Lili and yourself, Hoifung, have raised. And that’s really core for how we think about, you know, AI in this space of biology and how we actually operationalize that. So, it’s not sufficient to just develop competent models that can learn over protein sequences or protein structures or other biological modalities. What we really care about in this next phase is how do we bring those models to translation to unlock real biological discovery. And so as a step toward doing this, we’re really excited about a long-term partnership that we have with the Broad Institute of MIT and Harvard, specifically focusing on cancer cell biology and this notion of, how do we think about cell states in cancer beyond just the genomic measurements of point mutations and alterations that are in DNA? But how to actually use AI to learn representations of cells as a whole and how they interact with other local influences in their microenvironment. And so, we’ve launched a a research collaboration with the Broad Institute called Project Ex Vivo that really kind of exemplifies this theme of bidirectionality. And in fact, we have a dedicated lab group and wet lab space at the Broad Institute where we can actually, as computationalists and AI scientists, go in and inform the design of experiments that are generating data and also validating the predictions of our models that we’re developing. And I think that speaks to this core challenge for this next phase of AI for biology, of how do we actually build synergistic relationships that are truly bidirectional, and use that as a catalyst to actually bring these modeling efforts to the biological lab. So that’s something that’s at a high level really exciting and really top of mind for me.
Poon: Awesome. That’s really fascinating because you’re basically, you know, bridging the dry and the wet lab, right. So you’re basically having the infinity loop start kicking in, having a discovery cycle kind of like really going on steroids. That’s really fascinating. So along that same line, Lili, you mentioned earlier, the aspect that you’ve been focusing on is a lot of this kind of remote sensing opportunity, right, and often to some extent addressing some of the gap that conventional clinical data collection may or may not have at high density. So, kind of like similar question to you is like, what do you see as some of the major bottlenecks and what does what do you see as some of the key learning?
Qiu: Sure, Hoifung. Yeah, continuous health monitoring has lots of advantages, but it does introduce significant challenges. Here, I will quickly go over five main challenges that we’ve been looking at. First is achieving ease of use. Hospital-grade monitoring devices often require professional medical staff to operate, but in-home devices need to be very intuitive and user friendly so that elderly people such as grandma and grandpa can operate them independently. To address that, we are developing very simple wearable sensing, such as earphone sensing that requires minimal user intervention. Second, we need to achieve high precision and robustness. In-home health monitoring devices are typically designed with cost-efficiency in mind, and ideally, they need to support noninvasive sensing. This makes it very challenging to achieve with high accuracy and robustness. To enhance reliability, we are exploring hardware and software co-design. On the hardware side, we are developing advanced metasurface to dramatically enhance the sensing resolution. Metasurface, consist of many sub-wavelength cells like shown here. And these elements are designed to manipulate waves in ways that conventional material cannot. By carefully designing the geometry and material properties and spatial arrangement, we can enable metasurface to have very high flexible control over wavefront shaping and achieve high-resolution sensing. On the software side, we are developing advanced machine learning algorithms to support multimodality data. Third is to increase the sensing range and support mobility. Users expect a continuous monitoring regardless of their location, so sensing should continue to work even when users are moving around throughout their environment. So we developed a metasurface-based approach to enhance the sensing range so that a user can be sensed even when they are far away, or even when they are covered with blankets. Fourth is the mental state sensing and training. Continuous sensing does not just track vital signs, but also provides real time insights into a person’s cognitive and emotional well-being. We are developing an approach to track a user’s mental state. Meanwhile, we are also developing a large language model-based cognitive training tool that draws on each user’s daily experience to reinforce episodic memory. This ensures these exercises not only feel relevant but also engaging. We demoed our cognitive training tool on last year’s World Alzheimer’s Day and receive great feedback. And we think continuous at-home monitoring and tailored intervention can potentially improve cognitive care. Last but not least, achieving timely processing and energy efficiency. Because this continuous monitoring can generate a large volume of data that must be processed in real time on a user’s device. So our team is actively working on edge computing and lightweight modeling to achieve low latency and enhance energy efficiency.
Poon: Wow. That’s fascinating really. Thanks so much for sharing. When I personally think about continual monitoring, the first thing that comes to mind is like the continual is the glucose monitoring, right? For diabetes patients. But what you touch on, some of the cool sci-fi stuff, like this metasurface. Like people maybe eventually can wear a shirt, right, to keep monitoring and also go beyond just metabolic but also some of the mental and other, that that’s really fascinating. So, I want to continue toward more and more clinical application. So Carlo, you earlier mentioned about sort of like really two themes, right. So one is when we think about precision health, one of the marquee poster child? is cancer. So you mentioned some of the application side like tumor board trial matchings and so forth. You also mentioned another part which is on the technological front, right. Like how do we go about some of this kind of like, emerging modality, like pathology, but also even spatial omics? And also you seem to also touch on some of what Ava and Matt alluded to also eventually how do you pull those multilingual right, multi-model together. And so again, similar question is like what do you see in both fronts, application and technology? What are some of the key challenges that you see that really keep you up at night?
And what is some of your learning over the years?
Bifulco: Yeah. So super exciting to hear from Ava and from Lili on the progress that you can make in basic science and, and also, on the, not on the patient, but at a personal level in in collection of large data sets and so forth. On the clinical side, things are a little bit more challenging because we have a lot of overhead coming from the complexity of patient care, regulatory frameworks and so forth. So the progress is, you know, is great, but not always as great as I think as it could be. There are some sweet spots still that I feel are, you know, where we can move rapidly. And I think those have to do with the areas that are really of translation. So thinking of clinical trials is a setting where we can move very rapidly and really make an impact. Biomarkers is another area where we can do to same, real-world evidence is another area where things can move super rapidly. Having said that, you know, I’m super optimistic about the impact of AI. Even in routine patient care. I mean, I don’t want to sound too worried about this. I think there’s a very bright future ahead of us, and almost inevitable. But I just wanted to acknowledge that there are some complexities and some challenges in bringing this technologies to fruition in a conventional setting. And all of those can be, you know, overcome. But they will require a big effort at multiple levels.
Poon: Awesome. And and so now back to you, Matt. So as you, mentioned. You have this really amazing, almost like panorama kind of wheel, from academic to industries. And now really leading our scientific effort on our product health and life sciences product division. So, what do you see? Kind of like coming to see from all these angles, from foundational research, to a partnership to productization. What do you see ultimately, how do we address some of this last mile? Some of like what Carlo mentions. How do we really make the I count for patient care.
Lungren: Yeah. I was just kind of just reflecting on some of the comments around the, the incredible innovations that have that have been going on. This is just obviously, as you know, a small sample of some of the tremendous work that we see. And then I do my clinical time and I walk by a fax machine in my office, right. So there is a gap. There’s no question about it. Carlo knows very well. And, you know, on a macro scale you can see it in sort of the conferences. You can go to NeurIPS and see some incredible things, and then you can go to the American Medical Association conferences. There is a divide, but I think that’s closing. And I’m extremely optimistic. And part of it is, you know, you look at successful applications of AI to solve a clinical problem. And I’ll just use one example that much of the audience may be familiar with, which, you know, when you think about what are the immediate needs, like before we can get to the future state, the things we want to see happen, we have to solve the problem. We have to put out the initial fire. And the fire, of course, is really burnout, right? We have a crisis obviously, in the U.S., but certainly, this is this is an issue, I think, across the world. And clinicians have too much to do, too much complexity to sort of handle. And they’re looking for some time back, if I’m being honest. And so, you know, when I look at some of these technologies, I sort of say, we’re going to save time before we save lives. And the DAX Copilot solution, if you if you’ve heard of it, it sounds very simple on its face. Maybe you wouldn’t, you know, present this as the keynote at a computer science conference, but yet it’s solving an incredible problem. And what’s interesting about it is by saving that doctor some time, you’re also generating kind of a comfort level with, hey, this AI solution is actually making my life better. But it’s also to the to the point about the last mile. It’s also in the last mile. And from that place can we build out there? So, you know, just to use an example, Lili’s talking about some biometrics. Well, there’s voice data that comes from that interaction that then gets transcribed into a note. Well, there’s voice biometrics that we all are starting to become very familiar with. Well, now I can start to add that in. And now there’s almost a foothold right into the real clinical world. And you’re building from a place that physicians are kind of congregating around or at least familiar with. And so, I’m extremely bullish. And that’s outside of just the fact that maybe more so than ever, you see seen the New England Journal has a new journal about AI. That is a huge sign to me that there is an absolute, you know, Cambrian explosion of interest from the clinical side, not just sort of in the technology, but just how do we use this and how do we learn about applications for this? So, maybe more so than ever, I feel like that that divide is starting to really close.
Poon: Yeah, that that’s a really amazing insight. You know, you mentioned about, DAX Copilot. Right. And but also this whole feel about kind of ambient intelligence, right? That it feels like, you know, even just a couple of years ago. This feels super kind of sci-fi novel concept that you could have patient and doctor talking. And then actually the computer actually take care of the notetaking, right? And maybe even start to alert, you know, some of the things that maybe the doctor may have forgotten and so forth. Even, again, even a couple of years ago, felt so sci-fi. But now actually, DAX has, right, like, as you mentioned, has already taken so much, kind of, [INAUDIBLE]. But also there is a lot of other kind of similar undertaking that really testify to how this notion that like the genAI revolution can really move things much faster, right? So that that is so, so exciting. And also, I really love what you bring up about like, save time before saving life. So by starting from sort of like productivity again, then eventually we could really get to the even the creativity gain. So that that’s super, super exciting. So, I think we can talk, you know, for hours and hours, but I think we, we have, some final chance to start to kind of elicit some of the other insights from you guys. And I think this will be a very fun run. So we’ll basically ask each of you to make a prediction for the future? Like what might happen in five years, right? And so, Ava, can we start from you?
Amini: I have the hard job of going first for this question (laughing)
Poon: Or the easy one (laughing)
Ava: I think, you know, honestly, we’re at this incredible moment of a true inflection point in our capabilities with technologies like AI and it’s really impossible to predict exactly what’s going to happen next. But I have my vision and ideas about what that landscape could look like. And so right now, we’ve seen breakthroughs in our ability to use the power of generative AI and foundational models to learn over these individual biological modalities. So, for example, protein sequences like our work with EvoDiff or pathology data like GigaPath and Virchow and other biological modalities that are coming to the forefront. As an immediate step, I think we’re going to see a shift in bringing this information together, right? To be able to reason across the scales and the hierarchy of biology, from the level of individual molecules to cells to tissues to patients, and try to, you know, put this information together from a modeling perspective and also from an application perspective. And then I think looking forward to a few years down the line hopefully that leads to this vision that you raised Hoifung of how do we actually bridge that iterative loop between the experimental world and the computational world, such that we have AIs that can help us propose new hypotheses and go test them in the lab. And I think that’s something that I’m optimistic to see in five years. And hopefully that leads to drugs, therapies that are discovered or designed from scratch by AI and are put into pre-clinical or clinical testing. And I think that’s a very real possibility to think about. And then finally, to close, you know, I think this panel is really awesome because we have these different perspectives of, you know, someone thinking about fundamental research at the biological scale, like myself and Lili, with the perspectives on, you know, how do we interact with humans in their real lives and in kind of a cultural sense and the clinical partnership with Providence and, you know, the scaling perspective that Matt brings. And my ultimate vision is that five years from now, we have a way as Microsoft to come together and put these pieces, like bring these pieces together to life, to really create solutions at scale and that’s pretty high level. But I think that we are empowered to do that. And that’s what I’m really excited about.
Poon: That’s amazing. Just to echo some of your points about this kind of multi-scale learning, right, to bridge between different scales, by biological mechanisms and so far, but also really to, actually closing this discovery and translation loop. So that that’s super exciting. So, Lili, you highlight did this really important part to how can we fill in some of this CAB modality that conventionally may not be collected. So I’m curious, if you’ve thought about in five years. What do you see could actually happen. Will we all have metasurface vests? (Laughing)
Qiu: Yeah. That’s our hope. First of all, I’d like to express my deep appreciation for the opportunity to learn from Hoifung, Ava, Carlo and Matt, your perspective. For continuous health monitoring, what I see is, it’s more than just a technology advancement. It’s a paradigm shift in healthcare. So it means that healthcare is no longer constrained inside a hospital. It’s everywhere. No matter where we are, every step we move, every eye movement we make, every breath we take, every heartbeat we strike, every brainwave signal we generate is being sensed and analyzed to understand our health condition. And what we envision for the future is there will be no more travel, no more waiting room, no more frequent needle pricks, just timely virtual interactions with doctors empowered by advanced sensing and AI. And this shift is not only meaning convenience, but also hope. So with the aid of continuous monitoring, even minor physiological frustration, such as a slight rise in blood pressure or subtle changes in heart rhythm or blood glucose level, can be detected before they escalate into life threatening crises. By continuously tracking each patient’s state, a doctor can tailor their treatments and adapt immediately, rather than waiting on tests only taken at occasional hospital visits.
So our vision is to put advanced diagnostic and treatment at people’s fingertips so that they can use at home to make healthcare more proactive, personalized and powerful than ever before.
Poon: Oh, that’s amazing. Thanks. Thanks so much, Lili. when I was posing this question about in five years, I was also thinking about how people constantly make this kind of joke about in five years from now. And then, obviously this day, one of the hot topics would be AGI. Right. So apparently probably we will get AGI before five years. But when we think about, let’s say curing cancer, right? So like I joke with my team that let, let’s try to solve cancer before we get one. And so Carlo, I’m curious about what’s your take in five years? Like how close are we to start fundamentally, understanding, you know, cracking some of the cancer mechanisms? Will we be there in five years?
Bifulco: Yeah. Great question. And also hard act to follow, Ava and Lili’s, vision. But I don’t know how close we are going to be in fully understanding cancer, but I feel very confident that we will look back to our current stage as a dark age. Because I do think the impact of AI is going to be transformational. That applies to both biology as we heard before from Ava, you know, I do think we are currently generating so much data, and the capability of generating is so large that we need AI to actually manage that. But it also goes, you know, back to what Lili said. I do think in the future, AI will interact with people before they go to see a doctor. From a medical perspective, actually ideally not just medically, it’s health. It will really drive their health and their behaviors from a health point of view. In terms of medicine itself, I do think that we will reach a state where physicians will not be allowed to see patients without a companion, an AI companion of some point. I don’t know if that’s going to happen in five years, but I do think is going to be inevitable at some point. And lastly, you know, vis a vis Matt, you know, radiologists, pathologists. I think pathology and radiology are going to fuse and merge. I’m not sure is going to be great news for the radiology. But I think all the imaging modalities are going to be one single thing. And AI is going to mediate that interaction with the physicians and that kind of landscape.
Poon: Yeah. Fascinating. And, and I think also, as Ava mentioned earlier, aside from this existing conventional imaging modality, there are also a lot of these emerging, like measuring cell states and everything, and then coupling all of them together. It’s kind of like incredible, like a microscope to some extent. A virtual microscope. But also incredibly challenging because like you said, the high dimensionality, the noise and everything and, and, and really sort of tailor make for AI to hopefully help. And so, the should we say billion dollar question, the trillion dollar question, how can we really make this kind of viable, to put this technology to eventually democratizing this, high-quality healthcare to everyone? Matt, we really want to lean on you with all your diverse perspective. What do you see would be happening in five years?
Lungren: Well, I mean, I can at least predict that we won’t have fax machines anymore.
Poon: You sure? (laughing)
Lungren: I’m willing to bet on that one. Five years is hard, right? In this current environment. We’re seeing some pretty surprising acceleration in sort of the general purpose technologies, I think you’ve heard from some of the speakers. So I mean, we are clearly, I think advancing science at a pace that at least I haven’t seen in my career in my career in the last 20 years. So, with that being said, you know, I think that there’s a couple things I want to I really want to emphasize, what Lili said. I had a mentor once that said, you know, he was the chair of a very prestigious department and he always would go to these ribbon-cutting ceremonies when we’re celebrating the opening of hospitals. And he always said, why are we doing that? Like we should be celebrating the closing of hospitals. Like we really should be advancing care to the point where a lot of these things are preventative. We’re taking care of these things outside of the, you know, the four walls of the hospital where errors can occur. There’s all kinds of potential risks that go beyond just the conditions you have and obviously the complexity of that. So I do celebrate that. And I think that, you know, some of the steps in terms of how we scale to that, to that point, I mean, one of them is, you know, it’s not sexy to talk about, but it’s data. And we’ve done a tremendous job, I think, for the most part, in starting to collect data and digitize data and to be able to manipulate in its individual modalities. But what we haven’t done a great job of is really leveraging that data to, to drive insights even with what we have. This is beyond just sort of, you know, new data and new discovery. And I think, you know, in five years, I would be surprised if we don’t look back and really facepalm at the idea that we were leaving all this data on the table sort in archives and then only using it in episodic, you know, taking care of a patient and, and not using for insights such as real world evidence. How can I find similar patients, from practice-based evidence. Right. And leveraging that decision-making from, from real-world data. I think that is, something that, you know, we’re really close to at least at least seeing the possibility or pathway towards when it comes to some of the, I think the, the connection between the biological space and I think is Lili said, you know, the wearable space. I think there’s some connections there that, you know, I would hazard to guess are really going to start to come into fruition. I’m thinking specifically about, you know, less invasive monitoring over time. But then also tying that to, you know, biomarkers that may predict early development of cancer and other chronic diseases. And then to Carlo’s point, hey, I’d be been great with partnering up with you, in the reading room. We both love dark rooms and, you know, we can work together to take care of patients in our dark rooms. I’m good with that.
Bifulco: I’m looking forward.
Poon: Well, that that’s incredible. One thing that, Matt, you mentioned about that data, right? Like, I kind of learned from John Halamka from Mayo. He mentioned that actually, by some estimates, even today, 3 billion people in the globe already have many of their medical records digitized. But when you think about it what are we using those data? Right now it’s mostly like some immediate diagnosis, some billing. Right. Then they’re locked in the attics gathering dust. So to your point, like, like actually that’s a super exciting opportunity from all of you that is like, you know, genAI could potentially be able to understand the language of those data, multi-model longitudinal data. And then harnessing them to really for good use. So this is amazing and really thankful for all the panelists. Today I think we heard from like all the way from, you know, biomolecules and proteins and all the way to continuous sensing to, you know, patients and also from research and productization. So I personally learned quite a bit and, and I, I feel even more energized. So thank you very much. Thanks to everyone for listening.
So thank you.
Lungren: Thank you.
Qiu: Thank you.
Amini: Thank you.
Bifulco: Thank you.
- Series:
- Microsoft Research Forum
- Date:
- Affiliation:
- Microsoft Research, Providence Genomics
-
-
Hoifung Poon
General Manager, Health Futures
-
Ava Amini
Principal Researcher
-
Lili Qiu
Assistant Managing Director
-
Matthew Lungren
Chief Scientific Officer, Microsoft Health and Life Sciences
-
-
-
Series: Microsoft Research Forum
-
-
-
-
-
-
-
-
-
A generative model of biology for in-silico experimentation and discovery
Speakers:- Kevin Yang
-
-
-
-
-
-
AutoGen Update: Complex Tasks and Agents
Speakers:- Adam Fourney
-
MatterGen: A Generative Model for Materials Design
Speakers:- Tian Xie
-
-
Insights into the Challenges and Opportunities of Large Multi-Modal Models for Blind and Low Vision Users: CLIP
Speakers:- Daniela Massiceti
-
Research Forum 3 | Panel: Generative AI for Global Impact: Challenges and Opportunities
Speakers:- Jacki O'Neill,
- Tanuja Ganu,
- Sunayana Sitaram
-
-
-
-
-
-
What's new in AutoGen?
Speakers:- Chi Wang