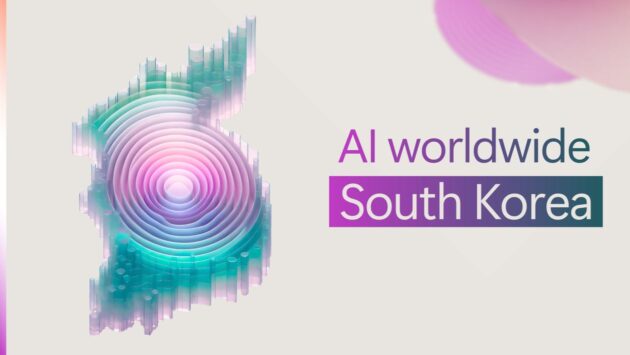
How South Korea is building an AI-powered future for everyone
At the Microsoft AI Tour in Seoul, Korean companies demonstrated how AI is moving beyond efficiency gains to become a true growth engine.
The rapid advancement of AI has ushered in an era of unprecedented capabilities, with large language models (LLMs) at the forefront of this revolution. These powerful AI systems have demonstrated remarkable abilities in natural language processing, generation, and understanding. However, as LLMs continue to grow in size and complexity, new challenges have emerged, including the need for more accurate, relevant, and contextual responses.
Enter retrieval augmented generation (RAG)—an innovative approach that seamlessly integrates information retrieval with text generation. This powerful combination of retrieval and generation has the potential to revolutionize applications from customer service chatbots to intelligent research assistants.
Let’s briefly uncover the future of AI-powered language understanding and generation through the lens of retrieval augmented generation.
Here are five key features and benefits that will help you understand RAG better.
RAG models rely on external knowledge bases to retrieve real-time and relevant information before generating responses. LLMs were trained at a specific time and on a specific set of data. RAG allows for responses to be grounded on current and additional data rather than solely depending on the model’s training set.
Benefit: RAG-based systems are particularly effective when the data required is constantly changing and being updated. By incorporating real-time data, RAG patterns expand the breadth of what can be accomplished with an application, including live customer support, travel planning, or claims processing.
For example, in a customer support scenario, a RAG-enabled system can quickly retrieve relevant and accurate product specifications, troubleshooting guides, or customer’s purchase history, allowing users to resolve their issues efficiently. This capability is crucial in customer-support applications—where accuracy is paramount—because it not only enhances the user experience and fosters trust but also encourages the continued use of the AI system, helping to increase customer loyalty and retention.
RAG excels in providing contextually rich responses by retrieving data that is specifically relevant to the user’s query. This is achieved through sophisticated retrieval algorithms that identify the most pertinent documents or data snippets from a vast, disparate data set.1
Benefit: By leveraging contextual information, RAG enables AI systems to generate responses that are tailored to the specific needs and preferences of users. RAG also enables organizations to maintain data privacy, versus retraining a model owned by a separate entity, allowing data to remain where it lives. This is beneficial in scenarios such as legal advice or technical support.
For example, if an employee asks about their company’s policy on remote work, RAG can pull the latest internal documents that outline those policies, ensuring that the response is not only accurate but is also directly applicable to the employee’s context. This level of contextual awareness enhances the user experience, making interactions with AI systems more meaningful and effective.
Explore how Microsoft AI can transform your organization
What are hallucinations?
Learn moreRAG allows for controlled information flow, finely tuning the balance between retrieved facts and generated content to maintain coherence while minimizing fabrications. Many RAG implementations offer transparent source attribution—citing references for retrieved information and adding accountability—which are both crucial for responsible AI practices. This auditability not only improves user confidence but also aligns with regulatory requirements in many industries, where accountability and traceability are essential.
Benefit: RAG boosts trust levels and significantly improves the accuracy and reliability of AI-generated content, thus helping to reduce risks in high-stakes domains like legal, healthcare, and finance. This leads to increased efficiency in information retrieval and decision-making processes, as users spend less time fact-checking or correcting AI outputs.2
For example, consider a financial advisor research assistant powered by RAG technology. When asked about recent Security and Exchange Commission filings regarding a publicly traded company in the United States from EDGAR, the commission’s online database, the AI system retrieves information from the latest annual reports, proxy statements, foreign investment disclosures, and other relevant documents filed by the corporation. The RAG model then generates a comprehensive summary, citing specific documents and their publication dates. This not only provides the researcher with current, accurate information they can trust, but also offers clear references for further investigation—significantly accelerating the research process while maintaining high standards of accuracy.
RAG allows organizations to use existing data and knowledge bases without extensive retraining of LLMs. This is achieved by augmenting the input to the model with relevant retrieved data rather than requiring the model to learn from scratch.
Benefit: This approach significantly reduces the costs associated with developing and maintaining AI systems. Organizations can deploy RAG-enabled applications more quickly and efficiently, as they do not need to invest heavily in training large models on proprietary data.3
For example, consider a small-but-rapidly growing e-commerce company specializing in eco-friendly garden supplies. As they grow, they face the challenge of efficiently managing and utilizing their expanding knowledge base without increasing operational costs. If a customer inquires about the best fertilizer for a specific plant, the RAG system can quickly retrieve and synthesize information from product descriptions, usage guidelines, plant zone specifications, and customer reviews to provide a tailored response.
In this way, RAG technology allows the business to leverage its existing product documentation, customer FAQs, and a scalable internal knowledge base where the RAG system expands with the business, without the cost or need for extensive AI model training or constant updates. By providing accurate and contextually sensitive responses, the RAG system reduces customer frustration and potential returns—indirectly saving costs associated with customer churn and product returns.
RAG helps boost user productivity by enabling users to access precise, contextually relevant data quickly by effectively combining information retrieval with generative AI.4
Benefit: This streamlined approach reduces the time spent on data gathering and analysis, allowing decision-makers to focus on actionable insights and teams to automate time-consuming tasks.
For example, KPMG built ComplyAI, a compliance checker, wherein employees submit client documents and request that the application review them. The app reviews the documents and flags any legal standards or compliance requirements, then sends the analysis to the user who originally set up the task. The app handles the review and analysis, saving the requestor time and effort. Thus, the app allows the user to ramp up on the topic or issue in question much faster without requiring them to be a legal expert.
As a result, users are more likely to perceive the AI application as a helpful and integral part of their daily tasks, whether in a professional or personal context.
In summary, by leveraging the vast knowledge stored in external sources, RAG enhances the capabilities of LLMs, including improved accuracy, contextual relevance, reduced hallucinations, cost-effectiveness, and improved auditability. These features collectively contribute to the development of more reliable and efficient AI applications across various sectors. RAG-enhanced systems also help empower smaller-sized businesses to compete effectively with larger competitors while managing their growth in a cost-effective manner, without the need to hire additional staff or for substantial AI model updates and retraining.
AI agents are changing the way we work
Explore moreTo get started, use the following resources to start building RAG applications with Azure AI Foundry and use them with agents built using Microsoft Copilot Studio.
Organizations across industries are leveraging Azure AI and Microsoft Copilot capabilities to drive growth, increase productivity, and create value-added experiences.
We’re committed to helping organizations use and build AI that is trustworthy, meaning it is secure, private, and safe. We bring best practices and learnings from decades of researching and building AI products at scale to provide industry-leading commitments and capabilities that span our three pillars of security, privacy, and safety. Trustworthy AI is only possible when you combine our commitments, such as our Secure Future Initiative and our Responsible AI principles, with our product capabilities to unlock AI transformation with confidence.
1DataCamp, How to Improve RAG Performance: 5 Key Techniques with Examples, 2024.
2 Lewis, P., Retrieval-Augmented Generation for Knowledge-Intensive NLP Tasks, 2020.
3 Castro, P., Announcing cost-effective RAG at scale with Azure AI Search, Microsoft, 2024.
4 Hikov, A. and Murphy, L., Information retrieval from textual data: Harnessing large language models, retrieval augmented generation and prompt engineering, Ingenta Connect, Spring 2024.